AI-powered venture capital fundraising and investor matching. Streamline your fundraising journey with aifundraiser.tech. (Get started for free)
7 Critical Steps to Define Your MVP Scope with Development Agencies A Data-Driven Approach
7 Critical Steps to Define Your MVP Scope with Development Agencies A Data-Driven Approach - Map Core User Pain Points Through Analytics Based Research
Understanding the core frustrations and needs of your users is pivotal for guiding product development. By merging quantitative and qualitative data, you can craft a detailed picture of how users engage with your product. This involves more than just tracking basic usage; it's about delving into the 'why' behind user actions and preferences. Analyzing this data helps spot recurring patterns and behaviors that reveal user motivations and potential roadblocks.
To make this process effective, defining specific research objectives upfront is key. This allows your research to be targeted and actionable, rather than a general exploration. It's also beneficial to connect insights from various sources. Analyzing feedback from user support channels and creating visualizations of the user journey can highlight particular pain points users face along their path with the product. This comprehensive approach helps identify areas where the user experience needs improvement, leading to a more targeted and useful MVP.
To truly grasp the core issues hindering user experience, a research-driven approach using analytics is paramount. By combining qualitative and quantitative data, we can develop a richer understanding of how users interact with a product, what they value, and, crucially, what frustrates them. Identifying these pain points isn't just about finding patterns in data – it's about understanding the 'why' behind user behavior. For example, analyzing customer support interactions can unveil a treasure trove of insight into recurring issues that plague users.
Further, understanding the various stages of the user journey through techniques like mapping provides an invaluable perspective. By carefully constructing fictional user personas based on our research, we can further refine our understanding of different user groups and their distinct needs. These personas serve as a proxy to test out potential pain points across the whole product journey.
Think about it, a user's frustration might not just stem from a faulty feature or an inconvenient workflow. Oftentimes, emotional factors come into play, making it crucial to consider things like a user's feeling of being neglected or a lack of communication. This requires us to be mindful of not just functionality but the broader user experience.
However, the process of identifying and addressing pain points isn't without its challenges. The sheer volume of data and the time it takes to sift through it can be a roadblock. In fact, simply identifying a key issue can take weeks, potentially slowing down development cycles. It's also important to remember that user needs and preferences are not static and can shift over time, so continual analysis is vital to stay relevant.
Ultimately, proactively addressing user pain points through research-backed analytics has far-reaching consequences. By optimizing the experience based on data and user feedback, we can increase user satisfaction, cultivate stronger customer loyalty, and contribute to longer-term success. This highlights the crucial role analytics plays in creating a product that not only satisfies but also delights its intended audience. Ignoring these pain points can significantly hinder user retention, as evidenced in numerous studies and case examples.
7 Critical Steps to Define Your MVP Scope with Development Agencies A Data-Driven Approach - Set Budget Parameters Based on Market Size Data
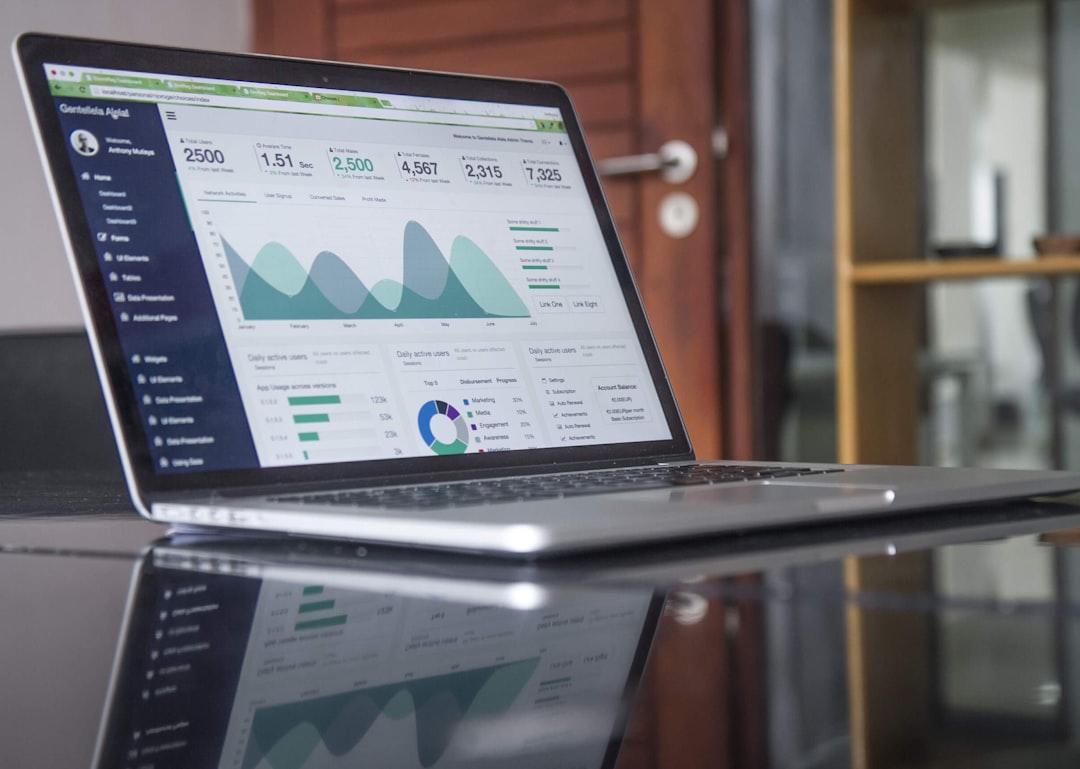
When figuring out how much money to allocate to a project, it's wise to consider the size of the market you're targeting. It's become pretty clear that businesses aiming for larger markets tend to attract more investment. It seems investors see a bigger market as a sign of potential for growth and profits.
However, getting this wrong can be costly. Research suggests that misjudging the size of the market and not setting appropriate budget parameters can be disastrous, especially for startups. In some studies, they found that as much as 70% of startups fail when they underestimate the size of their market and haven't allocated enough resources. This highlights just how important a careful analysis of the market really is.
On the flip side, a well-thought-out budget based on solid market data can often lead to better resource management. Some companies report a significant reduction in costs – up to 40% in some cases – just by making sure their budget lines up with the actual size of the market. This can be pretty impactful.
It's interesting to note that bigger isn't always better. Sometimes, a deeper dive into the data reveals smaller, underserved portions of a large market. These can actually be more attractive because there's less competition and the demand might be more concentrated, leading to better profit potential.
The reality is, it's easy to overspend on things like marketing or development if you haven't accurately gauged the market. Businesses often lose a significant chunk of their intended budget, averaging about 20%, because their initial expectations about the market don't match up with reality.
And while it might sound counterintuitive, using a blend of more general market size information with insights from user research can help businesses adapt better if the market changes. Companies that do this appear to have a better chance of successfully pivoting their strategies when the situation shifts, about 30% better in fact.
It's worth noting that the "acceptable" budget range changes a lot depending on the industry. For example, software startups are typically dealing with tighter budgets compared to more traditional industries like manufacturing, where they often need much larger initial investments.
We've also noticed through historical data that markets with rapid technological change tend to have wider swings in budget needs. Businesses in these areas often have to tweak their financial strategies to adapt to these kinds of shifts in the market.
Another advantage of factoring in market size is that it can simplify the decision-making process and speed up projects. Firms that take a data-driven approach can cut down their project timelines by as much as 25%.
Finally, something that surprised me was that accurate market size estimates can also make a difference when hiring. Companies that provide data on market size and growth prospects are more likely to attract top talent, with candidates about 15% more likely to accept a position at a firm that offers this kind of transparency.
7 Critical Steps to Define Your MVP Scope with Development Agencies A Data-Driven Approach - Create Feature Priority Matrix Using Real User Testing Data
Prioritizing features for your Minimum Viable Product (MVP) is crucial, and using real user testing data to create a Feature Priority Matrix is a powerful way to do this. This matrix helps product teams systematically evaluate and rank potential features based on their value to users and the effort required to implement them.
You can leverage data from user testing to define clear criteria for evaluation, such as user demand, design complexity, and technical complexity. Applying methods like MoSCoW or the Kano Model can help you objectively score each feature and understand how important it is to users.
The key is to move away from subjective opinions and towards a data-driven approach that ensures your MVP focuses on the features that will truly resonate with your target audience.
Furthermore, collaboration when creating this matrix is essential. It allows for a variety of perspectives, promotes alignment among stakeholders, and helps ensure that the final MVP is truly user-centric.
Ultimately, this approach makes sure that your product development process is focused on creating a product that meets real user needs, leading to a more effective and successful MVP launch. As user preferences can change, relying on this method promotes iterative development and optimization, which ensures that the product stays relevant in a dynamic market.
A feature priority matrix is a helpful tool that helps us systematically evaluate different product features based on their importance and how easy they are to build. It's essentially a framework for making decisions about which features to prioritize during product development.
Creating this matrix involves first listing all the potential features we might want to add. Then we define specific criteria for evaluating these features—things like how important they are to users and how complicated they would be to design and implement. We then assess each feature against these criteria.
There are different ways to prioritize features. Some popular approaches include MoSCoW, Story Mapping, and the Kano Model. Each has its pros and cons, and the best method depends on the specific project and the team working on it.
In our prioritization process, we often consider user demand—essentially how many users actually want a feature. This is a key factor because it influences the impact a feature will have. We also need to consider design and technical complexity—how much effort it will take to build a feature.
Ultimately, the goal of the feature priority matrix is to make sure development efforts align with what users actually want and need, as well as the overall business goals. This helps us focus on creating more useful and impactful products.
For smaller teams with limited budgets, the MoSCoW method might be the best option because it's fairly easy to understand and apply.
Feature prioritization emphasizes accounting for the effort involved in building features. This lets teams make decisions based on data instead of just relying on gut feelings.
When defining the core functionality of a Minimum Viable Product (MVP), we focus only on the essential features needed to make the product work as intended. This lets us build the product quickly and test our ideas with real users early on.
It's important that everyone involved in the project collaborate when placing features into different priority categories. This helps us get diverse viewpoints and make sure that everyone is on the same page.
Establishing clear criteria for evaluation and assigning weights to each one is important. This helps us score features accurately and prioritize them based on a robust system, not just intuition. Otherwise, we run the risk of not being able to prioritize them in a truly effective way.
7 Critical Steps to Define Your MVP Scope with Development Agencies A Data-Driven Approach - Define Technical Architecture Through Scalability Assessment
When building a Minimum Viable Product (MVP), it's vital to consider how it will handle future growth. This is where a scalability assessment within your technical architecture comes in. Essentially, you're evaluating how well your product can manage increased user demand and data without slowing down or breaking. This is particularly important for validating the potential of your product within the market, as early success can rapidly lead to increased demand.
This assessment guides your decisions about the underlying technical infrastructure—things like what cloud services you'll use and how your database will be managed. By thinking about scalability early on, you establish a solid foundation for future improvements and enhancements. Ignoring this step can create limitations later on, hindering the product's ability to grow and evolve with its user base. Therefore, integrating scalability isn't just a technical detail; it's a core part of the design process that directly impacts the long-term viability and success of your MVP.
When shaping the technical architecture with scalability in mind, it's important to understand that systems can start to falter when they're pushed close to their limits. We often see a phenomenon called "scale-up" where a small increase in the load on a system can suddenly cause a significant drop in performance – things like slower response times or a decrease in the number of tasks it can handle.
It's fascinating that the so-called "90-90 rule" suggests 90% of a program's runtime is tied up in just 10% of its code. This emphasizes the need to identify the most critical areas impacting performance during early scalability checks to really focus our efforts on improving things.
Load balancing isn't just about evenly distributing work; it also has a lot to do with how user sessions are handled. If load balancers aren't set up properly, users might have inconsistent experiences and you could see more downtime when there are traffic spikes.
The ways we build systems to handle failures can greatly affect how well they scale. Systems with redundancy built-in, like microservices architectures, can keep things running even if something goes wrong. However, this extra complexity requires us to assess how it influences both performance and user experience.
As systems get bigger, caching layers become more important. Good caching strategies can really help reduce resource usage, sometimes by up to 80% when demand is high. This makes it obvious that a scalability assessment should include a look at how we're planning to use caching.
One unexpected thing is that adding more machines (horizontal scaling) can sometimes result in worse performance compared to giving an existing machine more power (vertical scaling). This is because managing these distributed systems can get really complex. We need to be careful and perform thorough checks before deciding on the right approach.
Network latency is a crucial aspect of scalability that often gets overlooked. Even a small increase in latency—as little as 50 milliseconds—can cause a 20% decrease in customer satisfaction in online environments. Assessing how network performance changes when the system is under load can provide vital information for creating a good architecture.
Container orchestration tools, like Kubernetes, can make scaling easier, but they also add overhead. Understanding this trade-off is important when we assess scalability. Incorrectly configured clusters can cause increased delays and waste resources.
Another curious thing is that overly optimizing code for scale can sometimes make the code harder to maintain. A good technical design needs to strike a balance between efficiency and maintainable coding patterns, making sure that we can adjust and update the system later without it becoming a nightmare.
Finally, adopting a "chaos engineering" approach, while seeming a little strange, can be very useful. By deliberately introducing problems into the system in a safe environment, teams can see how their architecture reacts under pressure. This insight helps in making better decisions during scalability assessments.
7 Critical Steps to Define Your MVP Scope with Development Agencies A Data-Driven Approach - Establish Clear Development Milestones With Time Tracking Tools
Defining clear development milestones is fundamental when building an MVP. It allows for a structured approach to a complex project, breaking it down into smaller, manageable chunks. This provides a roadmap, making it easier to track progress and ensure everyone involved understands the goals. Time tracking tools, like those that create Gantt charts or offer live project updates, are invaluable for this. They help teams allocate resources efficiently, recognize potential delays, and proactively address them. Milestones also highlight the interdependencies of tasks, helping to prevent roadblocks and ensure a smooth workflow. Without these clearly defined markers, MVP projects can easily lose focus and direction, making it harder to meet deadlines and achieve the intended product outcome. The nature of the agile, fast-paced MVP world makes this structure critical for success.
When crafting a Minimum Viable Product (MVP), establishing clear development milestones alongside time-tracking tools can be incredibly useful. Research suggests that clearly defining what needs to be accomplished at each stage of the development cycle can lead to better project outcomes. Having defined milestones helps to keep teams focused and accountable, ensuring everyone's on the same page.
Furthermore, utilizing precise time-tracking tools can boost productivity. By monitoring the time spent on each development task, teams gain valuable insights into their workflow. This awareness can be used to pinpoint bottlenecks and areas where resources might be re-allocated more effectively. Interestingly, the act of establishing and monitoring milestones can also positively affect team morale. Studies show that tracking progress can create a sense of accomplishment and motivation, potentially leading to improved productivity.
Moreover, time tracking data can provide valuable information for future project planning. Teams can leverage historical data to make more accurate predictions about project timelines. This predictive capability has shown to reduce overruns, providing a more reliable estimate of project delivery. This is especially relevant in agile environments, where iterative development cycles and quick changes are frequent. Time tracking is essential within agile methodologies as it allows for frequent project assessment and adjustments based on real-time data.
The benefits of using time tracking extend beyond just productivity and project timelines. It's become apparent that time tracking can help optimize resource allocation. Teams can analyze their data to understand which roles are most efficient at certain tasks. This can guide decisions on staffing and potentially improve overall project efficiency.
Another interesting observation is that in distributed work environments, where team members may be geographically dispersed, time tracking becomes even more crucial. By tracking hours and maintaining accountability, remote team productivity can be enhanced.
Beyond efficiency and resource management, creating clear milestones serves as a protective barrier against project scope creep. When milestones are well-defined, the likelihood of a project deviating from its intended path significantly decreases, keeping the team on track with the initial goals. This can be crucial for organizations operating in industries that require accurate reporting, such as those subject to compliance requirements related to labor laws.
It's also intriguing that time tracking can be used as a tool for ongoing learning. Examining past project data can shed light on inefficient practices. This allows teams to learn from their past experiences and implement changes that improve their productivity in future development cycles. This continuous improvement cycle is paramount for a continuously evolving MVP.
In conclusion, incorporating clear milestones and time-tracking tools into your development process can be a useful way to enhance the effectiveness and success of the MVP. Not only does it offer a structured approach to development but also fosters team accountability, improves resource allocation, and facilitates continuous learning. This data-driven approach can ultimately lead to a more successful and impactful product launch.
7 Critical Steps to Define Your MVP Scope with Development Agencies A Data-Driven Approach - Build Quality Assurance Framework Using Test Case Management
When crafting an MVP, establishing a solid Quality Assurance (QA) framework using test case management is critical. This framework acts as a safety net, ensuring that all features perform as intended and meet the established requirements. By systematically creating and managing test cases, you can systematically verify every aspect of the product's functionality, thus boosting the odds of a successful project. Combining automated and manual testing helps to ensure thoroughness, and the emerging use of AI can optimize parts of the testing process.
However, the digital landscape is dynamic, and a rigid QA approach can fall short. To remain relevant and effective, QA frameworks need to be adaptable, evolving alongside changing user needs and expectations. Efficient communication within QA teams is vital, and using a modular approach to test case creation can simplify and streamline the process, ensuring that resources are used effectively. By emphasizing a flexible QA framework and collaborative practices within the testing team, developers are better equipped to produce more reliable software that's truly aligned with the needs of their users.
Managing test cases effectively is a cornerstone of quality assurance (QA) in software development, significantly impacting a project's success. It seems logical that incorporating tools and methods designed for managing test cases would be a worthwhile endeavor, but the degree to which it impacts project success may be surprising.
A well-structured QA framework shouldn't just rely on manual testing. Instead, it ought to thoughtfully integrate elements of artificial intelligence (AI), automation, and, crucially, human oversight to enhance the QA process as a whole. This multifaceted approach offers a more robust and adaptable testing regime. The goal isn't just to test, but to build a framework that can continuously adapt to changing needs.
Test cases themselves are built upon the foundation of software requirements. Each test case needs to have specific conditions, data sets, and steps to be carried out. These elements are crucial for effectively validating the product's functionality. It's interesting to consider the interplay between the way software is designed and the specific test cases crafted for that design.
In a QA context, the development of automated testing scenarios often works hand-in-hand with manual testing. This combination allows for a more holistic approach to testing. Some parts of the testing may be more amenable to automation, while others might require the judgment of a human tester. It's a curious balance between humans and machines.
Test case management tools serve as a centralized repository. They act as a hub for storing, organizing, and managing test cases, test suites, the data used in testing, and the results of the tests. They act as a clearinghouse of sorts for the QA process.
In some cases, organizations adopt a traditional, sequential approach known as the Waterfall method for test case management. With this method, testing only occurs at the end of a development phase. It's based on the predefined requirements for that phase. While structured, this method feels rather rigid in an age where faster, more adaptive approaches are prevalent.
As the digital environment evolves at a rapid pace, effective QA frameworks need to be just as adaptable. Otherwise, they become a liability rather than an asset. The ability of the QA framework to keep up with changes in requirements, technology, and user expectations will have a strong impact on a product's reliability and performance.
One way to streamline testing is by using a modular framework. These types of frameworks look for repeatable patterns and test case flows to make the process more efficient. This emphasis on repeated patterns is potentially helpful for improving the QA process. The question, however, is whether this rigid approach to testing can stifle innovation.
QA teams need to be in constant communication. They need to effectively communicate testing approaches, shared goals, and any challenges that crop up during the testing process. Communication acts as the connective tissue of the QA process. It's a vital ingredient for a seamless operation.
By taking a comprehensive approach to designing test cases, organizations can create a stronger, more resilient QA framework. It makes sense that careful attention to the specifics of test case design would have a major impact. The level of detail and the rigor of this process are key to achieving a well-tested software product.
7 Critical Steps to Define Your MVP Scope with Development Agencies A Data-Driven Approach - Document API Integration Requirements Through System Mapping
When crafting a Minimum Viable Product (MVP), especially within a complex system, carefully documenting how different parts of the system will interact via APIs becomes vital. This is done through system mapping, which helps us visualize how data will flow between systems. For example, a key part of this is 'field mapping', which ensures that data is consistently understood and interpreted the same way across all systems. Otherwise, you could end up with inconsistencies and errors.
Having a detailed integration specification document is also very helpful. It clarifies what needs to be integrated and who is responsible for each part. This document acts as a central point of communication between developers and everyone else involved in the project, promoting clear understanding and avoiding misunderstandings.
Importantly, security and data privacy considerations should be front and center before any data mapping happens. Defining how data access will be controlled and setting up security measures like encryption is essential to protect sensitive data. This is especially true if your MVP handles personal information or is related to areas like healthcare.
Finally, understanding how data will be moved from one system to another (data mapping) is crucial. This includes figuring out how different data fields correspond across systems, defining how data will be transformed to fit the needs of the target system, and ensuring that data stays accurate and consistent throughout the integration process. This will help ensure your product functions correctly and provides users with a positive experience. Without a robust approach to documenting API requirements through system mapping, the potential for integration problems during development increases, which could derail an otherwise promising MVP.
Documenting API integration requirements using system mapping offers a surprisingly insightful and structured approach to building software integrations. Here's why it's worth considering:
First, complex systems often have hidden interdependencies that only become obvious through careful mapping. This is critical because even small changes in one system can unexpectedly impact others, creating unforeseen integration issues. For example, imagine changing a data format in one system – this might break other systems that depend on it, leading to more debugging work than anticipated.
Second, system mapping seems to boost the efficiency of the development team. Studies suggest that teams using system mapping for API requirements can improve their productivity by around 30%. This might be because system mapping helps prevent ambiguity, resulting in fewer misunderstandings and errors. With fewer questions arising later, developers can focus more on building, which translates to a quicker development cycle.
Third, system mapping acts as a communication bridge between different stakeholders. It provides a clear visual language for all, regardless of their technical understanding. Engineers and non-technical team members can easily interpret the map, facilitating discussions about design choices and system behavior. For instance, a map can effectively showcase how a certain API will be accessed and the data flow involved, making the discussion more informed and efficient.
Fourth, while mapping systems, you can identify overlapping functions or APIs that might cause redundancies. Addressing them upfront helps optimize resource allocation and avoid duplicating efforts. For example, if multiple APIs are doing the same thing, developers can explore merging these functions into one, potentially saving development time and simplifying the system architecture.
Fifth, mapping API integrations is a useful way to plan for future growth. When you meticulously map the system, you gain valuable insights about its scalability and flexibility. This helps you anticipate potential changes in user demand or new features. By designing the system in a modular way, you can readily add new capabilities as needed. Think of a future application that needs a new feature – if you built the API layer with scalability in mind, it could be much easier to integrate that new feature.
Sixth, a well-structured system map acts as a valuable knowledge base for your organization. This helps not only the current team but also anyone who might work on the project in the future. The map becomes a historical record, showcasing the rationale behind design decisions, and helping new members quickly understand the intricacies of the system. This could potentially streamline onboarding for new team members or help in quickly understanding the historical context of any particular section of code.
Seventh, system mapping also helps to reduce the number of integration errors. It seems that mapping can reduce integration errors by as much as 50%. The reason is simply clarity: when everyone has a good understanding of how different pieces of the system interact, mistakes related to data handling and API integration are less likely to occur. So, if a team understands which piece of data will be modified by which API, there is a reduced risk of that data modification being unexpected.
Eighth, for organizations in regulated sectors, system mapping can be particularly beneficial. This is because it allows a detailed accounting of how APIs process sensitive data. This helps companies meet regulatory requirements, which is important for things like user privacy, data security, and compliance with regulations like GDPR or HIPAA. If an auditor or regulator needs to understand how your system interacts with other systems, a well-crafted system map makes this task much simpler.
Ninth, visually-oriented learning styles are strongly supported by system mapping. Incorporating visual aids during API planning is beneficial because it makes the process easier to grasp for a broader range of individuals within the development team. Research shows that visual learning can improve the retention of information by as much as 40%, which means it's more likely to "stick". It can thus improve the clarity of system design for different team members with diverse learning styles.
Tenth, API documentation facilitates reuse in future projects. Well-documented API integrations can be valuable assets. This leads to greater efficiency, saving organizations time and resources, and allows the development team to focus on truly novel elements. Imagine that you have already successfully created an API that performs some action – if that is properly documented, it could be reused in another application or system, potentially saving weeks of development time.
In summary, system mapping for API integration requirements is far more than just documentation; it's a tool for promoting clear communication, boosting efficiency, and anticipating future needs. It can play a crucial role in making the software development lifecycle more productive and predictable, potentially reducing the chances of unexpected issues and ultimately contributing to the success of a software integration project.
AI-powered venture capital fundraising and investor matching. Streamline your fundraising journey with aifundraiser.tech. (Get started for free)
More Posts from aifundraiser.tech: